Products
Our products are based on our own research achievements and have been continuously developed and improved over several years. With our products, we can automate individual work steps in your production, thereby saving valuable labor time. Safety is significantly enhanced, especially when handling heavy workpieces.
Vision guided robots - powered by AI
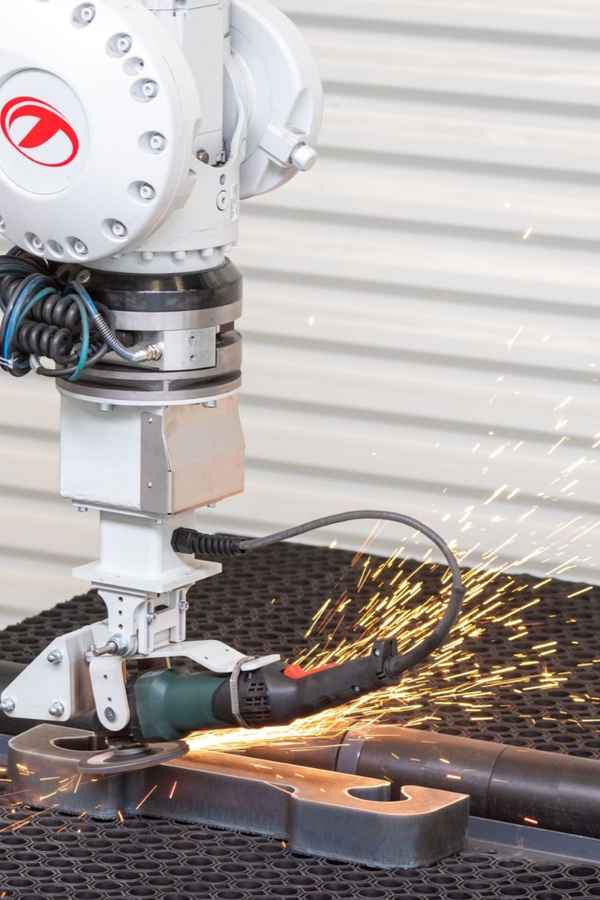
EasyGrinder
Grinding robot for oxyfuel and plasma-cut workpieces!
Read more
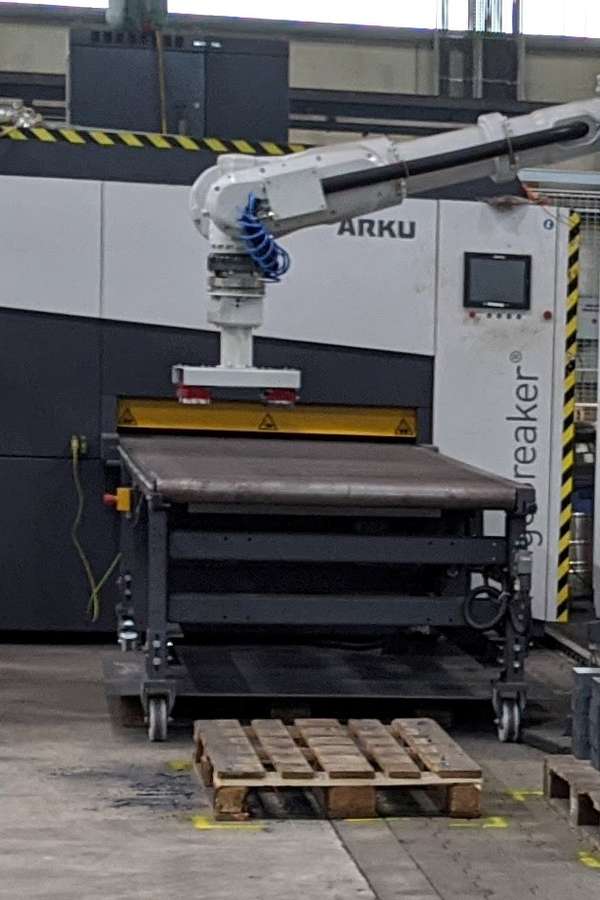
EasyDebur
Load and unload any brand of deburring machine with our robot - without programming
Reas More
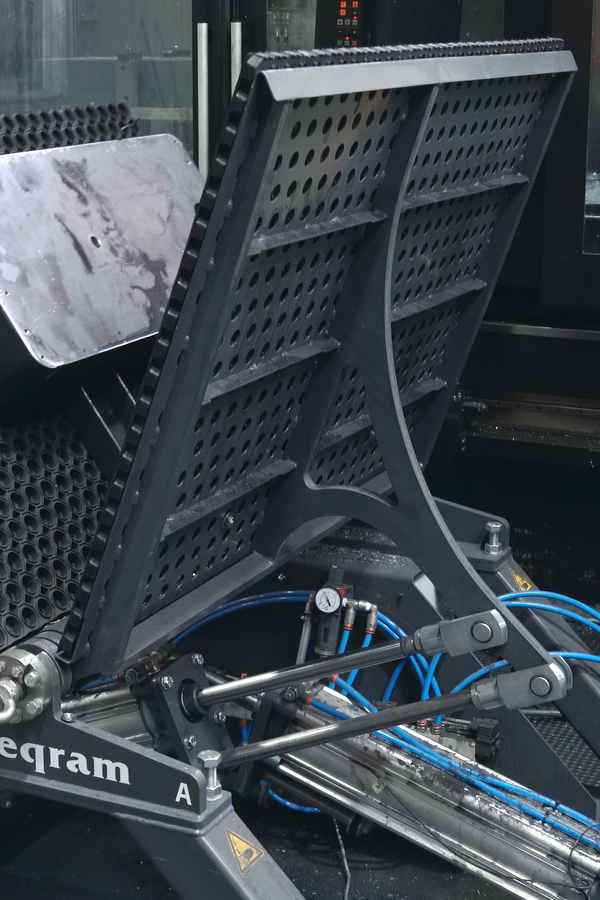
EasyFlipper
Turn or rotate heavy parts safely with our EasyFlipper, an innovative part rotating device
Read More
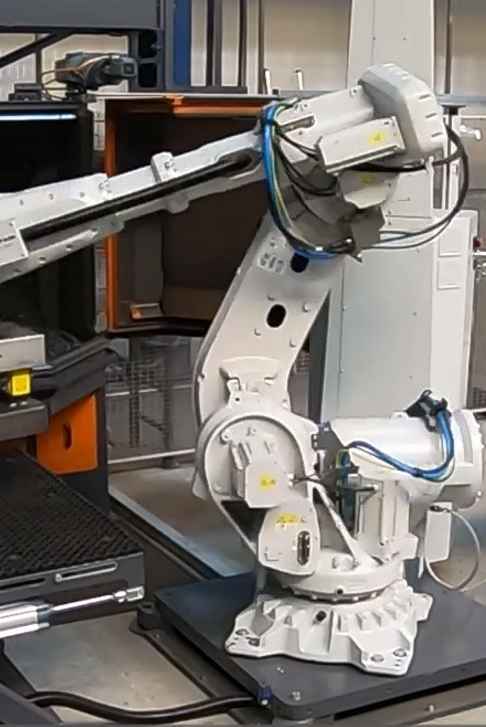
EasyShotblast
Load and unload shotblasting machines without programming with our EasyShotblast
Read More
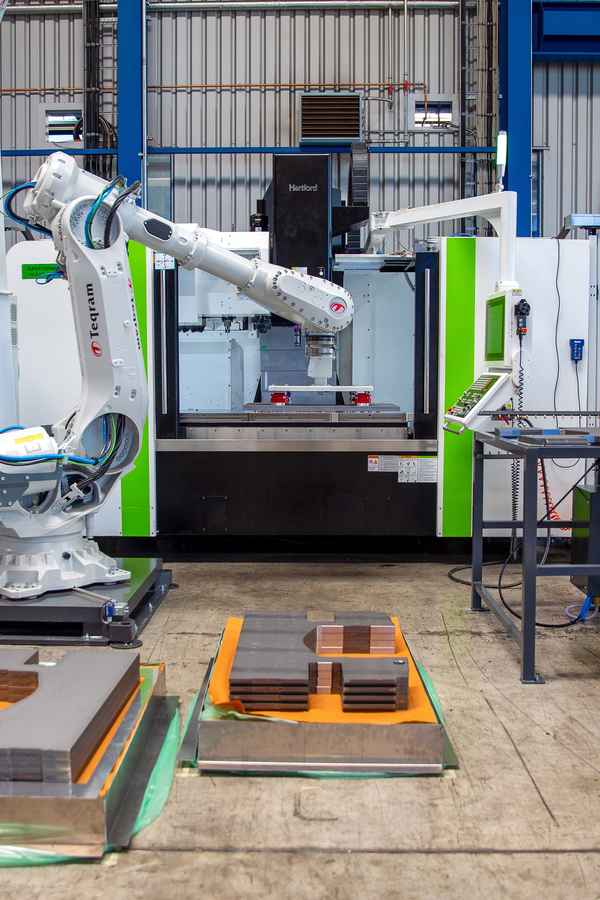
EasyMillDrill
Load and unload milling machines with workpieces up to 600 kg - without programming!
Read More
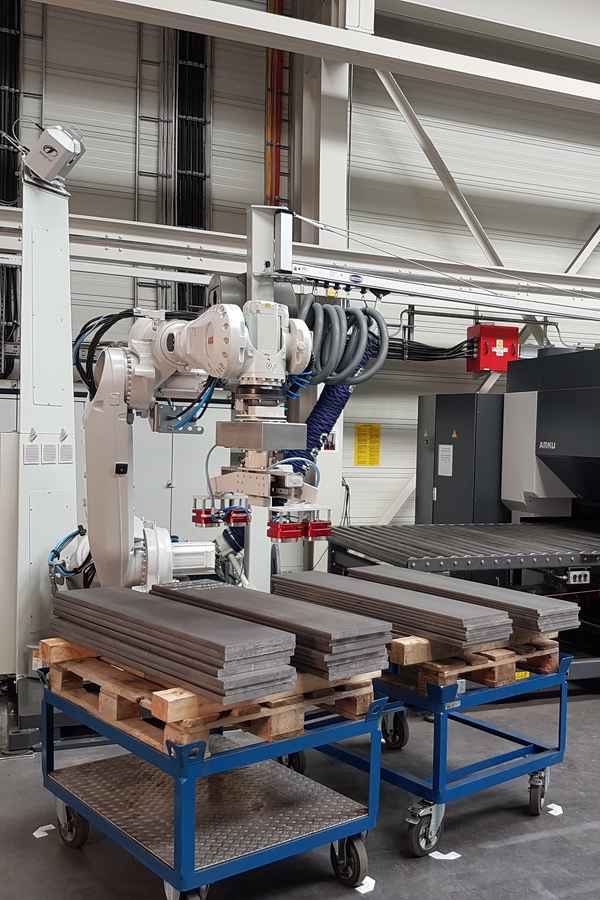
EasyLevel
Automated (un)loading of levelling machines for metal parts
Read More
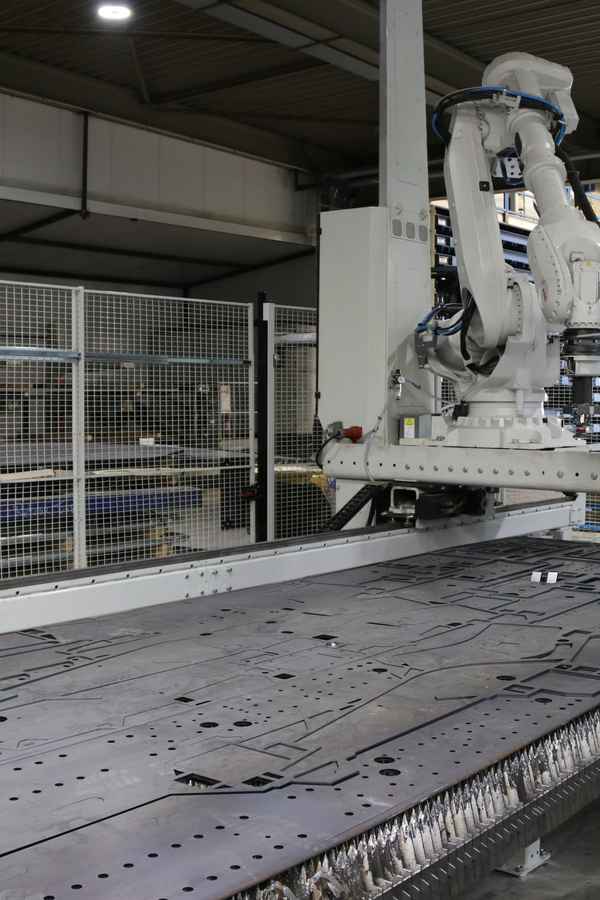
EasyUnpacker
Automatic loading of sheets for flatbed cutting machines including removal of scrap and unloading parts onto pallets.
Read More
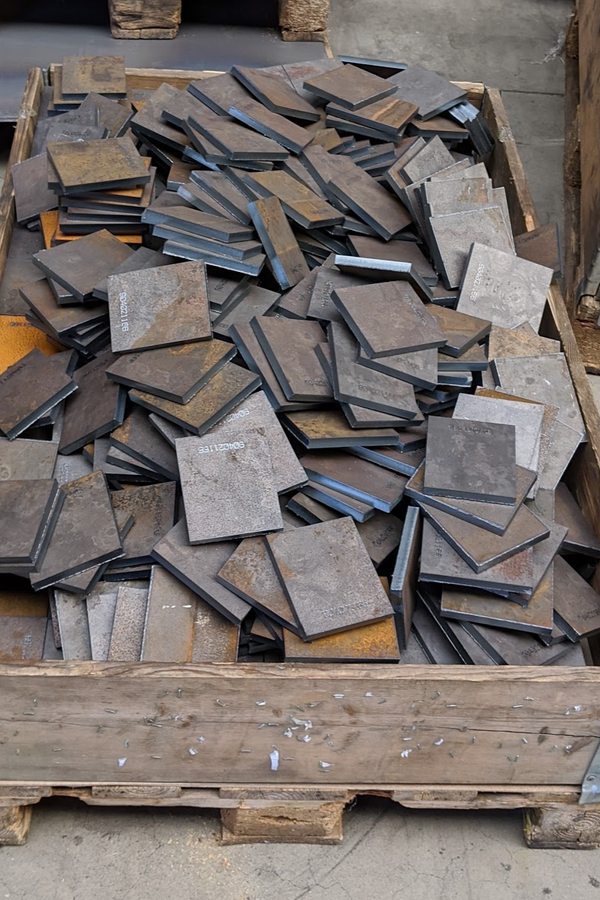
Bin Picking
The recognition of parts is the most important of flexibility in robot technology. It makes the difference between a 'dumb' pick and place unit and a smart and flexible solution.
Read More